Introduction
The field of data science has become one of the most sought-after career paths for ambitious students and working professionals in India. With the increase in the number of companies that rely on data to make decisions and shape strategies, the demand for skilled data scientists has grown rapidly. However, as much as popularity and growth may soar, so does the competition—and the potential to make mistakes that may go against your career interests. This book assists you in not making such mistakes and, therefore, allows you a better chance of getting hired and succeeding in a data science job. (8 Mistakes in Data Science Jobs | Best Tips to Avoid them)
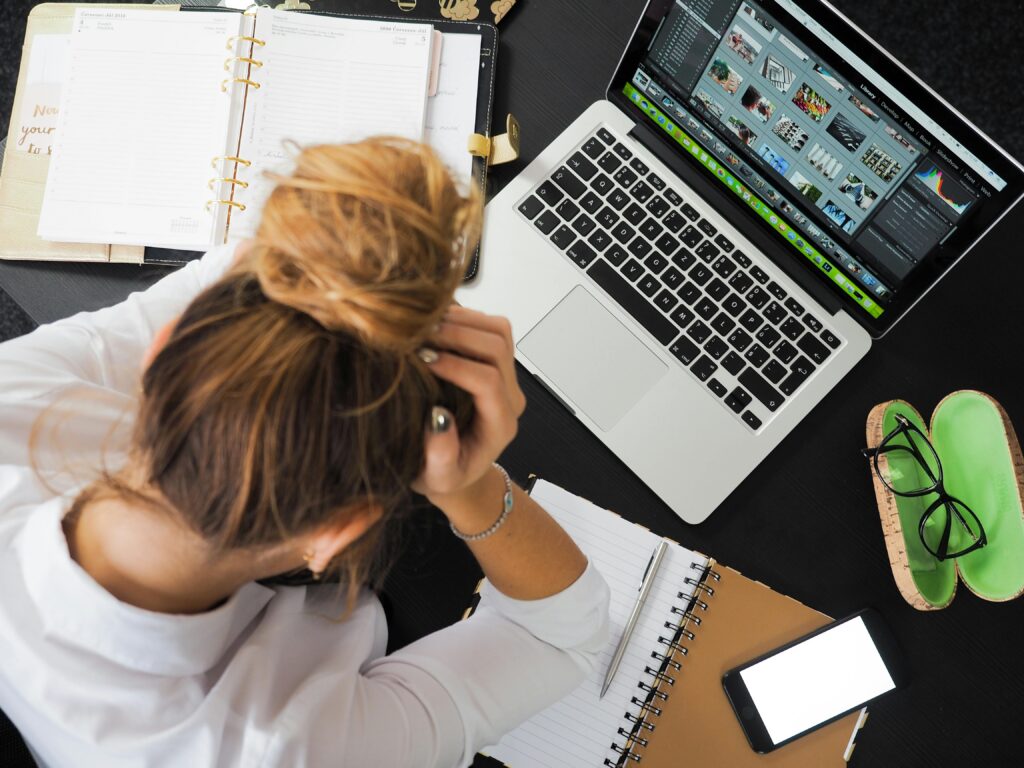
Jobs in the area of data science can be one of the most daunting of navigation whether by an amateur or for the career advancement of professionals. Among them are being overly focused on technical skills as well as underestimating business acumen. Without an explanation of these mistakes, several great data science professionals fail; others face them during their interview processes and miss opportunities on a dream job. That is what this blog is designed to do: cast a light on these so you are fully prepared for your interviews.
This ultimate guide will cover all the major blunders of data science jobs and interviews especially for Indians in 2023. We understand that from what recruiters look for to how you should effectively show your skills, we’ve got you covered. At the end of this post, you’ll know what the actions and strategies are for avoiding these blunders that will take you down a rewarding and successful path into a career in data science.
Mistakes in Data Science and How to Avoid Them.
1. Mis-interpreting the Data and Only Tool-based Work
Too often, data scientists err on the side of too many tools and forget the foundation: understanding of the data itself. Sorts of newbie enthusiasts rush forward to teach themselves programming languages like Python, R, SQL, but technical work of software is not how a strong data scientist varies from just an average practitioner. Knowing how to tell data tells you something-true something-is what creates the excellence of a solid data scientist.
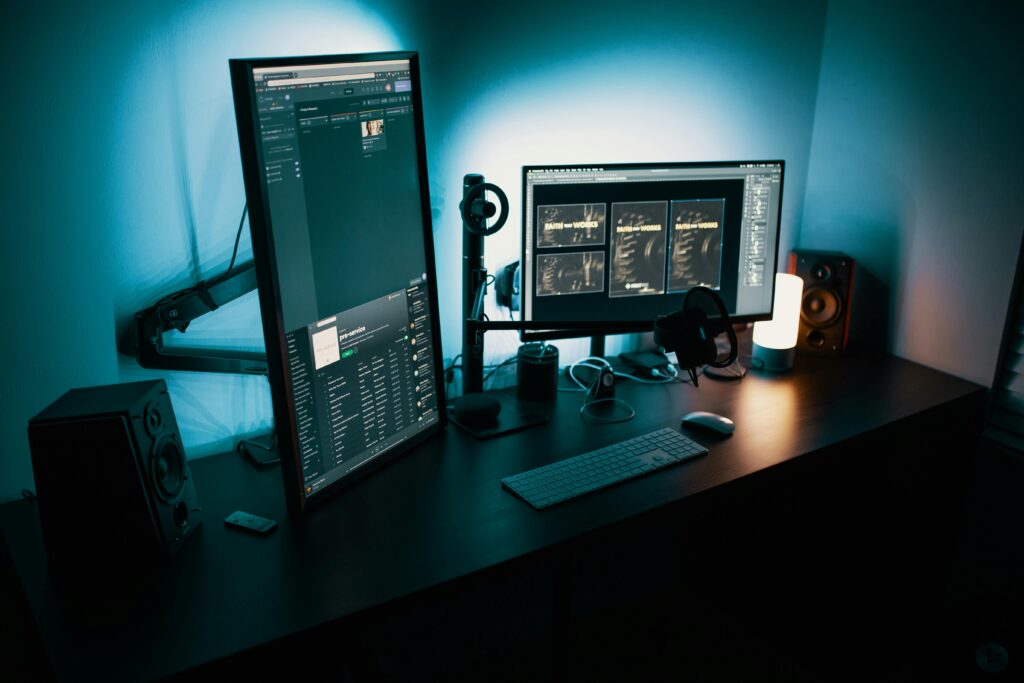
Focus should be on building up strength in statistics and interpretation of data. Remember that technology and tools are only tools, and the interpretation skills are always constant. And always ask yourself what the story is behind the data rather than just viewing some numbers or graphs.
2. Ignoring Domain Knowledge
Data science is not about numbers and algorithms, but about solving real-world problems. Without domain or industry-specific knowledge, you may end up with irrelevant insights or flawed analysis. For instance, if you work in the healthcare industry, you would need to know about industry regulations, patient privacy laws, and medical terminology. Without this domain understanding, your analysis will go off target, and both the quality of insights and your reputation will suffer.
Invest your time in understanding the industry that you are working in or planning to get into. You read the reports of the industry, attend webinars, and discuss with professionals in that area. This will make your approach to data science projects more holistic.(Mistakes in Data Science)
3. Over-reliance on pre-built models
While tempting to use the pre-built models and libraries, especially for beginners, this may prove to be an expensive mistake. Most professionals begin using libraries like Scikit-learn or TensorFlow without actually knowing what lies beneath the hood of these algorithms. As time progresses, this turns out to create a dependency on tools, which hampers the ability of a data scientist to think critically about the models he is applying.
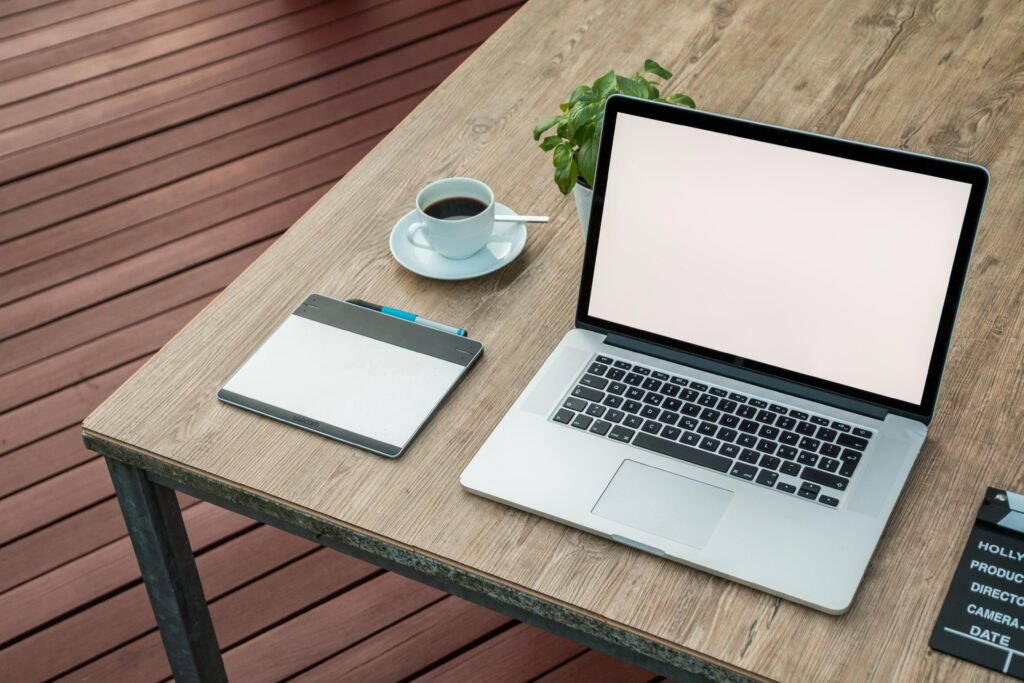
Solution: Instead of just loading the pre-built libraries, try to understand the mathematics and logic behind them. Start with simple models from scratch to have a good grip on the concept. Not only will it boost your confidence, but you’ll be prepared to face problems you didn’t anticipate.
4. Lack of display of soft skills in addition to technical skills while doing data science job interviews
One of the most common interview mistakes is only focusing on technical skills as if that is all what the recruiter cares about. Technical skills are important but recruiters in data science will also look for communication and teamwork and critical thinking. After all, data scientists explain their findings to non-technical teams and work with others across departments.
Solution: Practice speaking about complex technical topics in simple terms. Be prepared during the interview to share stories that illustrate your teamwork, problem-solving, and adaptability. This will demonstrate that you’re a skilled data scientist but also a valuable team player.
5. Failure to Ask Clarifying Questions
Often, candidates start diving into solving the problem in an interview without reading what it says. This sometimes produces answers that are half wrong and even gives a hint of over-confidence. Often, interviewers expect someone to ask clarifying questions before any attempted solution.
Solution: For any question, which can be a complex problem, take some time to first clarify the ambiguous points before answering. This shows one’s analytical mind and sharp eye for details-a prized skill in data science professionals.(Mistakes in Data Science)
6.Lack of practical project experience
Hands-on experience is probably the most important thing when it comes to data science. Most Indian students go in for interviews without having any hands-on projects to their name on their resume. Recruiters look for candidates who will demonstrate that skills garnered do indeed manifest in real-world practical challenges the candidate will be supposed to handle.
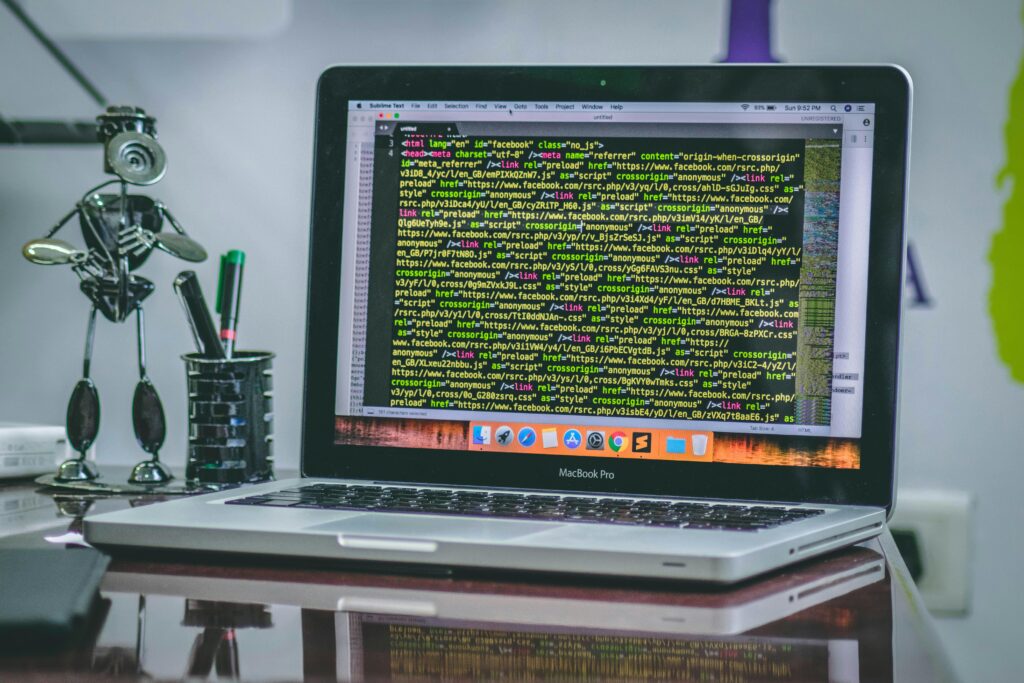
Solution: Internships, online challenges, and hackathons. For fresher, you can think of creating a portfolio in Kaggle competitions, open-source projects, or any of your own projects. A GitHub portfolio can be the difference while screening and distinguishing between him and others.
Mistakes Common to Indian Data Science Job Market
7.Excessive reliance on technical skills but a lack of business acumen
Job seekers with technical skills are always in demand in the Indian market for data science jobs, which is imperative but not sufficient by itself. Professionals in data science should understand the bottom-line impact of their work on the business.
The Indian market is increasingly looking for what have come to be known as “T-shaped” professionals – deep knowledge in one or two areas of technical disciplines but a breadth of business understanding.
Solution: Read business cases and understand how data science is applied across different industries. Consider how you can add value from a business perspective and be prepared to discuss this in interviews.
8. Overestimation of continuous learning importance
The data science field is a moving field where new techniques and tools come out every day with new frameworks. In most cases, professionals become lethargic because they easily get a job, but that finally leads to stagnation in one’s skills. (Mistakes in Data Science)
Indian firms look for candidates who want to learn continuously because their passion will ensure them to keep up with the latest from the industry.
Regular time should be spent on upskilling. Follow the data science blogs daily, go through some online courses, and stay active in the data science community every day. This will not only keep fresh skills but also make you more appealing to potential employers.
Conclusion
It, thus, leads to summary or avoiding all common mistakes when it comes to working as a data scientist would catapult one’s career especially if someone is considering Indian competition, and for the better one, would keep themselves one step ahead by sticking mainly to interpreting data and how to take hold of some core domain knowledge with extremely built soft skills.
Again, rectification of some basic interview mistakes along with being able to provide an understanding of working experience related to real world projects shall impact recruiters greatly.
For the latest data science insights and job opening updates, join our Telegram community. We update regularly on 10+ Telegram channels dedicated to the various niches of your career, plus free job notifications. This is useful for serious people who want a career in data science.
Lastly, to all those readers until here, we have a secret message to all of you: just comment below your Telegram handle and we will send a private invitation to the premium exclusive group where such a group of supportive people await you – for someone who cares about data science and is perhaps a career mentor. See you soon!
HOW AI AFFECTS THE ENVIRONMENT
Share the post with your friends