The Complete Guide to Fine-tuning DistilBERT for Indian Students and working professionals preparing for interviews or want to enhance career growth in AI, ML and data analytics We will explore the intricacies of fine-tuning DistilBERT for multiple tasks such as emotion classification, text classification, sentiment analysis, and multiclass text classification.
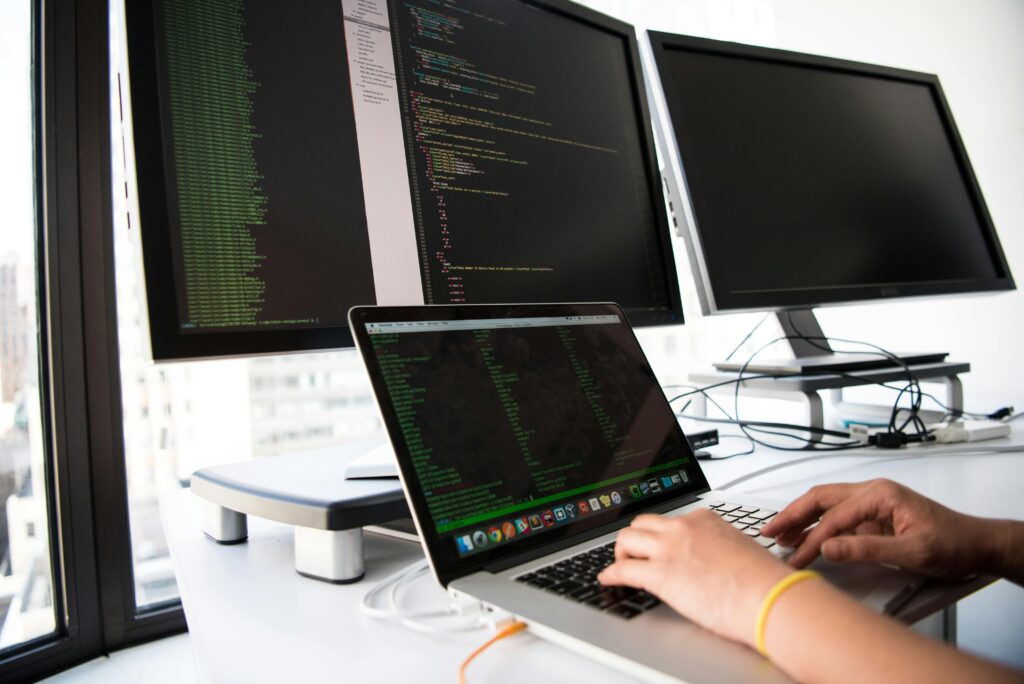
You will learn about this powerful technique for fine-tuning distilbert for text classification tasks and how to harness its capabilities to solve real-world problems. This will help you to be fully equipped with what you will face in Interviews — Basic to Advance. By the end, you will be confident and able to work on any fine-tuning distilbert, which will carry you out in the industry. We will discuss Fine-tuning DistilBERT through fine-tuning distilbert for emotion classification and: fine tuning distilbert, fine tuning distilbert for text classification, fine tuning distilbert for sentiment analysis, fine tuning distilbert for multiclass text classification, fine tuning distilbert for classification.
Well, in the AI-driven world today, it is very important to know the details of Fine-tuning DistillBERT. As an aspiring data scientist, machine learning engineer or data analytics career, this makes fine-tuning distilbert for the text classification a must-need skill. Note: This guide is specially written for Indian students/working professionals.
It simplifies intricate ideas into bite-sized pieces, offering real life examples and exercises to reinforce what you learn. This is a complete guide with this, you have a complete understanding of how to fine-tune distilbert and it surely gives you an edge in the job market. Familiarizing yourself with how to use distilbert kind of incorporates this two because you will have a solid foundational knowledge of implementing distilbert solution for various kinds of data problem you face.
Through this comprehensive guide to Fine-Tuning DistilBERT, we aim to enable you to appreciate and implement the intricacies of this vital procedure with confidence. For all the novices or the seasoned professionals, this detailed guide on fine-tuning distilbert provides a holistic understanding and enables you to excel in your career. You will learn everything you need to know about fine tuning distilbert, fine tuning distilbert for text classification and fine tuning distilbert for sentiment analysis in this ultimate guide.
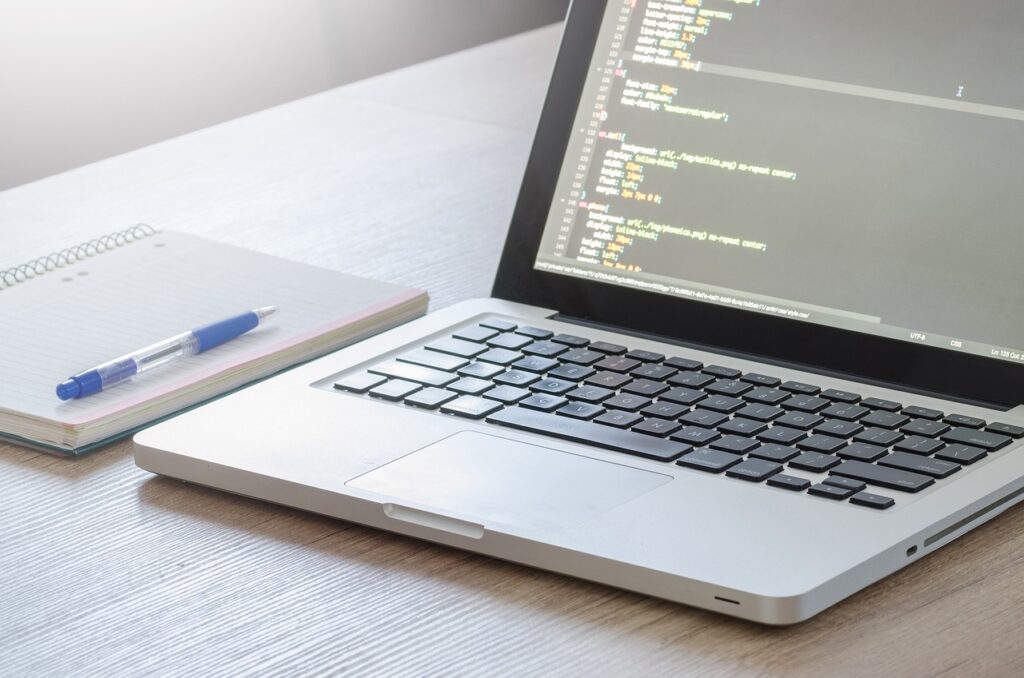
You will learn how to approach complex problems and leverage state-of-the-art computing resources to fine-tune DistilBERT for a large variety of text classification tasks — including multiclass text classification!
Fine-tuning DistilBERT
As a result, many developers and researchers turn to DistilBERT, a smaller and faster BERT obtained using knowledge distillation. Specializing distilBERT is a progression that adds additional specialization layers to fine-tune the previously fine tuned weights of the original Distilbert less data set for a deployment task or domain (e.g. sentiment classification) So you can take an existing model, fine-tune it, and use model transfers to work and you get free stuff.
This approach to fine-tuning distilbert allows you to train more complex models for your goals, quite easily and quickly. In addition, fine-tuning distilbert to classify text provides the model with specialized training that allows it to understand the intricate details and trends present within text data. This is important in many applications, from sentiment analysis to chatbot creation. Fine-tuning distilbert for sentiment analysis enables a better appreciation of the emotional tone in text, which is a highly valuable feature in the current digital landscape.
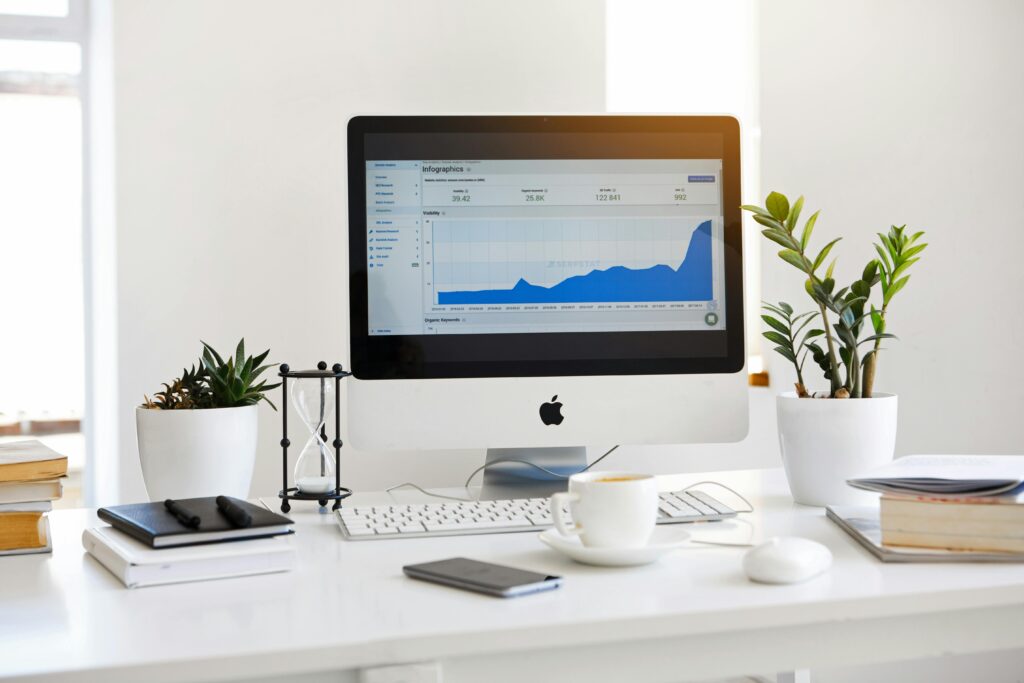
In this process, the most important steps include selecting a dataset, pre-processing it, selecting a fine-tuning distilbert model, training the model and finally evaluating the model. It’s all about the interaction between each step; knowing all of the steps is important, but knowing how each interacts is critical. In this post, we will take a closer look at these steps, using tangible examples and code snippets to illustrate how you can apply these ideas in your work.
We will go over different techniques you can use to turn down the knobs on your fine-tuning process, including hyperparameter tuning and the use of advanced techniques, all of which we will demonstrate using distilbert for sentiment analysis. This particular case focuses on optimizing distilbert for multicclass text classification which means you will be equipped to manage the challenges with complex cases. Add these, as there are more complex ways of treating many categories in your text classification and ensuring peak performance.
It is important to know the benefits of doing fine-tuning for DistilBERT. Not only that, you can memorize the details of the categories from DistilBERT with much less computational resources and less time and this guide is targeted for people who could not afford the latest gadgets or with limited logical reasoning.
The aim here is to know the particulars of fine tuning distilbert on classification and how tuning weights on distilbert helps you in solving text analysis use case. This holistic approach will help you understand the nitty-gritty underlying fine-tuning DISTILBERT to perform different text classification tasks.
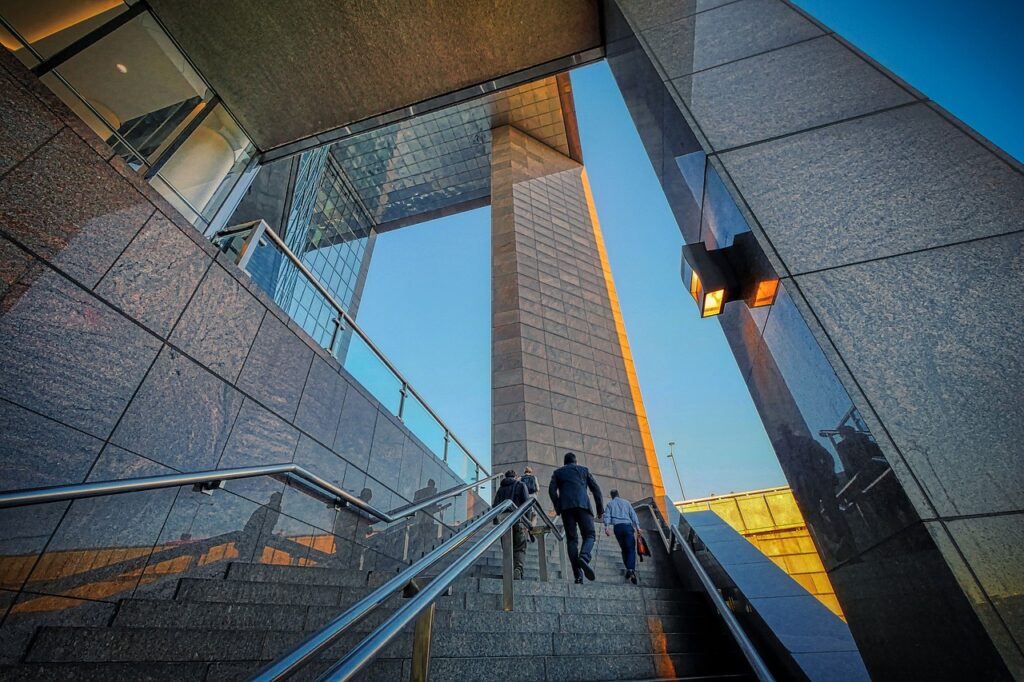
Important Considerations for Success
It is important to choose fine-tune distilbert hyperparameters. Hyperparameter tuning is a crucial part of optimizing the distilbert for text classification or sentiment analysis. It is important to know how these parameters affect the performance of the model.
With our step-by-step explanations and examples, you will learn not only how to use distilbert for your classification use cases but also how to tweak it and make the right decisions. In this step by step guide to fine-tuning distilbert, we will take you through the entire process, including the steps that are key for success.
Conclusion
Welcome to the end of this comprehensive, the best guide on fine-tuning DistilBERT. We hope this in-depth tutorial on fine-tuning distilbert has equipped you with adequate skills and knowledge. We really feel that this is the best guide to fine-tuning distilbert, all techniques are covered well. Again, practice consistently and develop an understanding of this powerful technique. You can indeed fine tune distilbert for classification problems and here we mounted all the tools you need to make it.
You made it to the end — now for a little secret: You deserve to join our top-tier, premium Telegram bubble. It is a community of learners and professionals like you who want to share and work together. Ask for an invite link by commenting down below with your Telegram channel and we’ll send you the invite.
Benefits like these will be at your fingertips when you join us on our premium Telegram community, and get things that you don’t! We will post about data science internships and other career opportunities regularly. Telegram channel Our community is a hub for discussions around the latest trends and upcoming opportunities in AI, ML, and data science. You will also be joining a community where you can ask questions, share your findings and learn from the experiences of others. You will be working in a collaborative and stimulating environment.
The supplementary Telegram channels that you could join offer different learning materials to help you grow in your learning and career path. You are given a plethora of tools in Data science, Machine Learning, Cyber Security, among others.
Our Telegram Group for Data Science Internships
We post data science internships for Indian students and professionals in our Telegram groups. It is a great way to build on your experience and get practical experience that can help make your resume shine and prepare you for a successful career in the field.