In this blog, I will share Top 5 Data Analytics Projects for Finance. In the ever-evolving landscape of finance, data analytics has become a cornerstone for informed decision-making and strategic planning. As we step into 2024, the intersection of finance and data science offers exciting possibilities for professionals seeking to leverage data-driven insights.
Here, we explore the best five data analytics projects for finance in 2024, providing a glimpse into the transformative power of analytics in the financial sector.
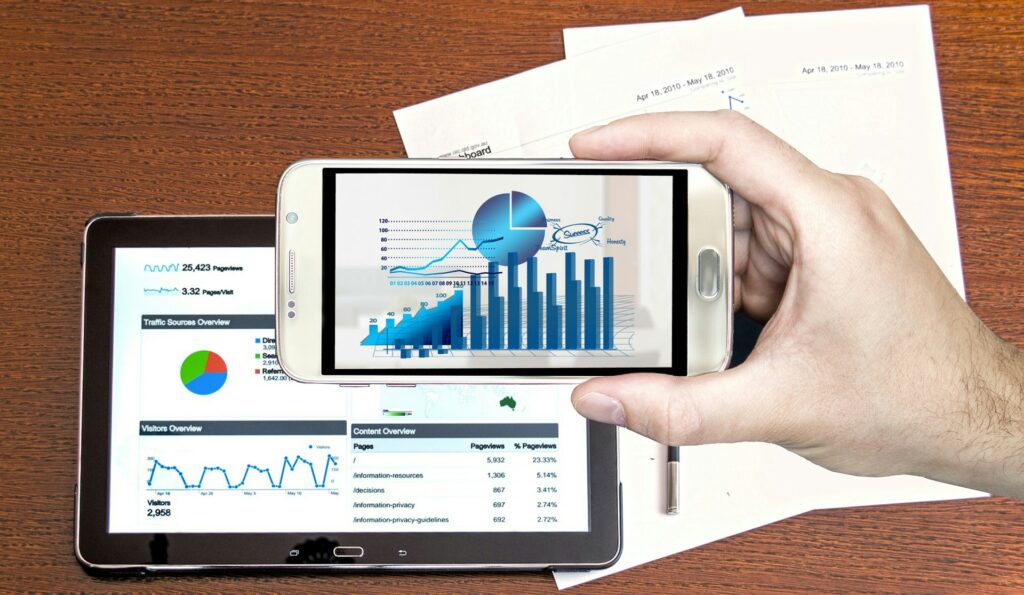
Predictive Modeling for Stock Prices
In the dynamic world of stock markets, predicting price movements is a real challenge. This is one of the interesting data analytics projects for finance that harnesses historical stock data, economic indicators, and market sentiment to forecast future prices.
Using machine learning algorithms, such as regression models or time series analysis, data professionals can build models that adapt to changing market conditions. From data extraction to real-time predictions, this project equips investors with invaluable tools to navigate the intricate web of financial markets.
Data Extraction:
- Collect historical stock prices, financial statements, and relevant economic indicators. You can use financial data APIs, such as Alpha Vantage or Yahoo Finance API, to fetch historical stock prices. Financial statements can be obtained from public financial reports, and economic indicators from sources like the Federal Reserve or World Bank.
Data Preprocessing:
- Clean the data by handling missing values, adjusting for stock splits, and calculating relevant financial ratios. Combine data from different sources and time periods for comprehensive analysis.
Feature Engineering:
- Create relevant features such as moving averages, technical indicators, and sentiment scores based on news articles or social media related to the stocks.
Model Training:
- Utilize machine learning algorithms, like regression models or time series models (e.g., ARIMA or LSTM), to train the predictive model. Split the data into training and testing sets to evaluate the model’s performance.
Evaluation and Deployment:
- Evaluate the model’s accuracy and performance using metrics like Mean Absolute Error (MAE) or Root Mean Squared Error (RMSE). Once satisfied, deploy the model to make real-time predictions on new data.
Credit Risk Analysis
The stability of financial institutions hinges on their ability to assess and manage credit risk effectively. This is one of the data analytics projects for finance that’s focused on credit risk analysis involves gathering, cleaning, and analyzing data on borrowers. By developing machine learning models that evaluate creditworthiness, financial organizations can make more informed lending decisions. This project not only enhances risk management but also contributes to the overall stability and sustainability of the financial system.
Data Collection:
- Gather data on borrowers, including financial statements, credit scores, payment history, and economic indicators. Use credit bureaus, financial institutions, and public financial reports as sources.
Data Cleaning and Integration:
- Clean and preprocess the data, handling missing values and outliers. Combine data from different sources to create a comprehensive dataset for analysis.
Feature Selection:
- Select relevant features such as debt-to-equity ratios, cash flow patterns, and credit scores. Use techniques like feature importance analysis to prioritize variables.
Model Development:
- Build a credit risk model using machine learning algorithms like logistic regression or decision trees. Train the model on historical data, considering both default and non-default cases.
Model Validation:
- Validate the model’s accuracy and performance using metrics like precision, recall, and the area under the ROC curve. Adjust the model parameters if needed.
Deployment:
- Deploy the credit risk model to assess the creditworthiness of new loan applications and continuously update the model with fresh data.
Algorithmic Trading Strategy
Algorithmic trading is at the forefront of financial innovation, and crafting a data analytics projects for finance around algorithmic trading strategies can revolutionize trading practices. From historical data retrieval to the implementation of automated trading systems, this project involves leveraging quantitative models and machine learning algorithms to optimize trading strategies. Traders can gain a competitive edge by harnessing the power of data analytics to make split-second decisions based on historical patterns and real-time market data.
Data Retrieval:
- Obtain historical market data, including price and volume information for relevant financial instruments. Data can be sourced from financial data providers or exchanges.
Data Cleaning and Feature Engineering:
- Clean the data, handling any irregularities or missing values. Create features such as moving averages, trading indicators, and volatility measures.
Strategy Development:
- Develop an algorithmic trading strategy based on technical indicators, trend analysis, or machine learning models. Backtest the strategy using historical data to assess its performance.
Optimization:
- Use optimization techniques to fine-tune the trading strategy parameters, maximizing returns and minimizing risks. This may involve adjusting indicators, time frames, or risk management rules.
Implementation and Automation:
- Implement the algorithmic trading strategy in a simulated or live environment. Integrate automation to execute trades based on predefined criteria.
Fraud Detection in Financial Transactions
With the rise of digital transactions, the threat of fraudulent activities looms large. A data analytics project centered on fraud detection in financial transactions is crucial for safeguarding financial assets. By employing machine learning algorithms to analyze transaction patterns and identify anomalies, financial institutions can detect and prevent fraudulent activities in real time. This project not only protects the interests of clients but also reinforces the trust and integrity of the financial system.
Data Collection:
- Gather data on financial transactions, including transaction amounts, timestamps, and user details. Include information on past fraud cases for model training.
Data Preprocessing:
- Clean the data, handling missing values and outliers. Create features such as transaction frequency, average transaction amounts, and geographical information.
Model Training:
- Utilize machine learning models, such as anomaly detection algorithms or neural networks, to train a fraud detection model. Use labeled data to distinguish between normal and fraudulent transactions.
Real-time Monitoring:
- Implement the model for real-time monitoring of financial transactions. Set up alerts for suspicious activities and continuously update the model to adapt to new fraud patterns.
Feedback Loop:
- Establish a feedback loop to retrain the model with new data and improve its accuracy over time. This ensures the model stays effective against evolving fraud techniques.
Portfolio Optimization: One of the best Data Analytics Projects for Finance
Constructing an optimal investment portfolio has been a perennial goal for finance professionals. In 2024, data analytics takes center stage in portfolio optimization projects. By collecting and analyzing data on various financial instruments, professionals can use mathematical optimization techniques to create portfolios that maximize returns for a given level of risk. This is one of the best data analytics projects for finance that allows investors to navigate the complexities of the financial markets with a strategic, data-driven approach to asset allocation and risk management.
Data Collection:
- Collect data on various financial instruments, including historical returns, volatility, and correlation coefficients. This data can be obtained from financial databases or APIs.
Risk Assessment:
- Assess the risk tolerance of the investor or organization. Define risk metrics and constraints that will guide the portfolio optimization process.
Mathematical Optimization:
- Utilize mathematical optimization techniques, such as the Markowitz Mean-Variance model, to construct an optimal portfolio that maximizes returns for a given level of risk.
Diversification Analysis:
- Analyze the diversification benefits of the portfolio, considering the correlation between different assets. Ensure that the portfolio is well-balanced and not overly concentrated in a particular asset class.
Performance Monitoring:
- Implement a system to monitor the performance of the optimized portfolio over time. Regularly rebalance the portfolio to maintain alignment with the investor’s goals and market conditions.
These detailed steps should provide a comprehensive overview of each project. Keep in mind that these projects involve a combination of data science, finance knowledge, and programming skills. The specific tools and technologies used may vary based on individual preferences and organizational requirements.
These five Data Analytics Projects for Finance offer a roadmap for professionals eager to embrace the transformative power of data in finance. From predicting stock prices to optimizing investment portfolios, the projects outlined here exemplify the Data Analytics Projects for Finance, heralding a future where insights derived from data lead the way to financial success. Embrace the data-driven revolution and unlock the full potential of finance in 2024.
You can combine all the data analytics projects for finance share above to create your own data portfolio.
You can access latest job opportunities by joining telegram channels: Job Opportunities & Data Analytics Jobs
Hope it helps 🙂
Share the post with your friends