Introduction
Data consistency is an essential data science, AI, and data analytics concept. You should know what data consistency is, how to detect and repair issues, and how various data consistency models work to boost your success in interviews and career advancement significantly. This is the ultimate guide; read this through.
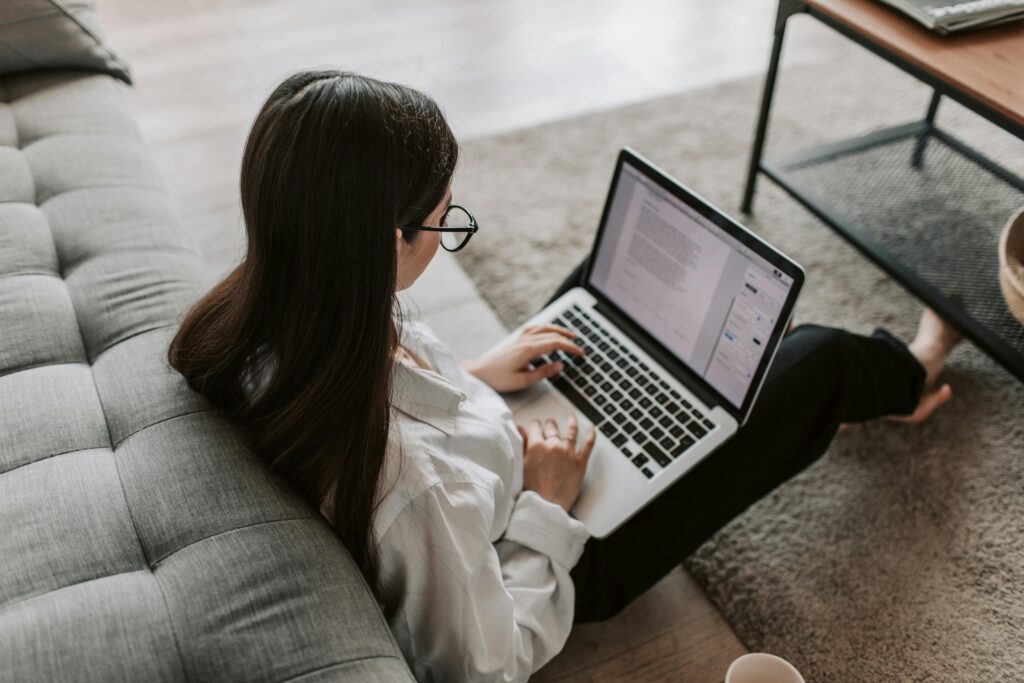
In today’s competitive job landscape, particularly for Indian students and working professionals searching for jobs in AI, ML, and data analytics, an excellent understanding of data consistency concepts is essential. This blog article will give you a complete and detailed overview of data consistency, and you’ll know why data consistency matters and how it contributes to your career. From what is data consistency to data consistency models, everything will be discussed here, and this will be the ideal go-to resource to answer interview questions on data consistency and integrity with confidence.
Data consistency, simply put, is that data within a database or data system is accurate, consistent, and shares the same meaning across applications and sources. It’s needed to produce credible insights and make the right predictions in AI and ML applications. Data consistency helps ensure that the data you’re employing for data analysis, model training, and decision-making is credible and accurate since inconsistent data will easily yield erroneous results. We will demystify how to detect and repair data consistency problems and how data consistency models can assist.
In this comprehensive guide to data consistency, we’ll delve deeper into the basic concepts and explore various data consistency models. Whether you are a seasoned data professional or a student starting your data science journey, this article will provide you with a proper introduction to this critical aspect of data handling and help you achieve your dreams.
Having knowledge about data consistency and how to effectively utilize it is invaluable in today’s data-driven era. Knowledge about data consistency is like having a treasure trove of opportunities, allowing you to navigate through difficult data environments with ease, facilitating better decision-making, and improving your chances in the highly competitive space of AI, ML, and Data Analytics.
Data Consistency Models: A Deep Dive
Data consistency models help manage and enforce the consistency and correctness of data in various parts of a system. Various models exist, each with its own advantages and disadvantages. Some of the most popular models are relational data consistency models, where data integrity constraints are defined using relationships among tables.
Knowledge about these models is crucial in handling inconsistent data efficiently, as inconsistent data can become a major factor in the outcome of projects. These models are particularly useful in the case of large databases and complex data structures commonly employed in AI and ML projects. Knowledge about data consistency models is required in handling data integrity and avoiding the errors arising due to inconsistent data, which in turn will help you improve your performance in interviews and contribute to your career growth.
Knowing how to use and implement these models effectively in real-world data science applications, whether in a small firm or a big corporation, is crucial. Knowing these concepts makes you a better team member and a thought leader in any firm. Various data consistency models apply to various requirements and complexities, so knowing the nuances is crucial to manage data efficiently. Whether dealing with structured data or unstructured data, data consistency principles are crucial to know.
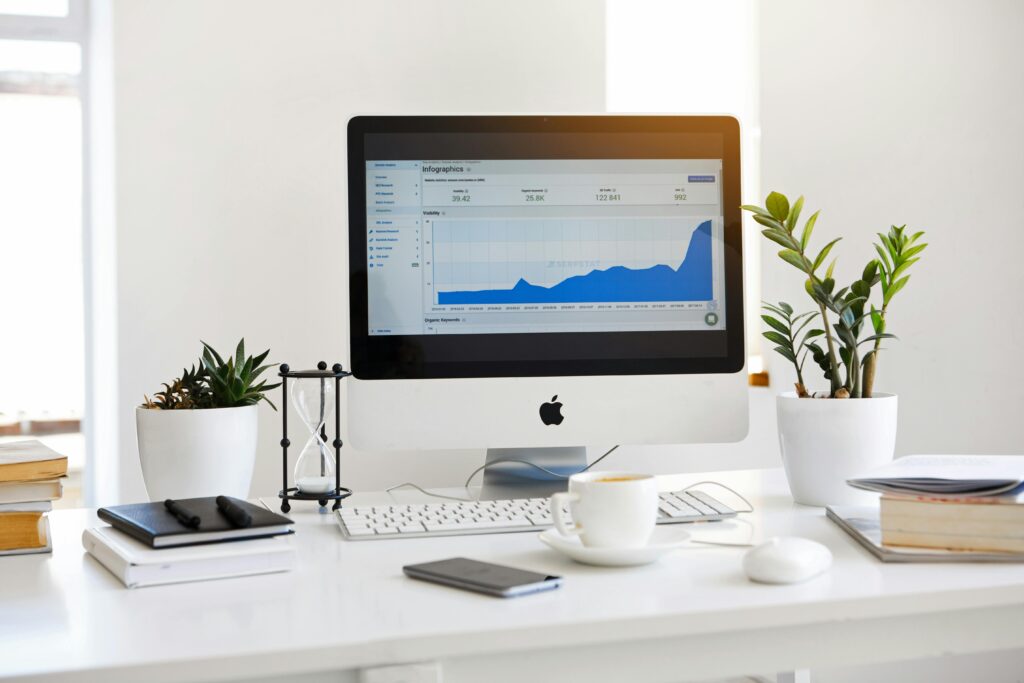
Let us discuss the practical application of data consistency, and examine examples. How do firms implement these models to deliver data integrity in their applications? How do you identify the signs of data inconsistency?
Data Consistency vs. Data Integrity: Key Differences
Though used interchangeably, data consistency and data integrity are different. Data integrity is concerned with the correctness and validity of discrete data points, whereas data consistency is concerned with data correctness and consistency between multiple data sources or data points. Suppose individual data points are correct, but the relationship between them is broken—this is where data consistency comes into play.
One of the key differences to know in your interview preparations is the precise focus of each. Data integrity ensures the accuracy and reliability of the data itself, whereas data consistency ensures the data is consistent throughout the entire dataset. This fine difference typically goes unnoticed, but knowing the nuance between the two in depth can be of immense assistance in your interview preparation.
In AI and ML projects, data consistency is crucial to train accurate and reliable models. Inconsistent data can lead to incorrect predictions, which can affect decision-making and business outcomes. Data integrity and consistency are a key differentiator between successful and unsuccessful data science projects.
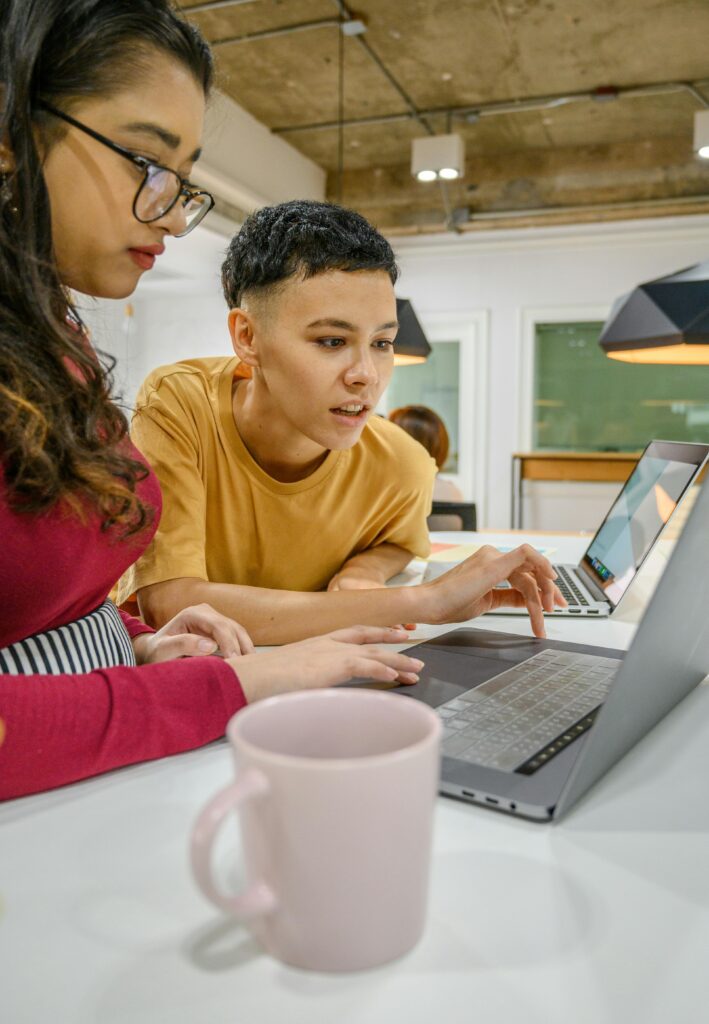
The Power of Practical Application: How to Impress Recruiters
Mastering data consistency is more than just book smarts. It’s about knowing how these concepts are used in practical applications. Think about how companies use data consistency in their applications. How do they ensure consistency of data across systems? What are the challenges they face? Knowing these real-world implications can significantly boost your interview answers.
To be able to apply these learnings in interviews says a lot about your practical smarts and sets you apart from other candidates. The ability to connect theory to practical examples will make your interview responses more interesting and stimulating.
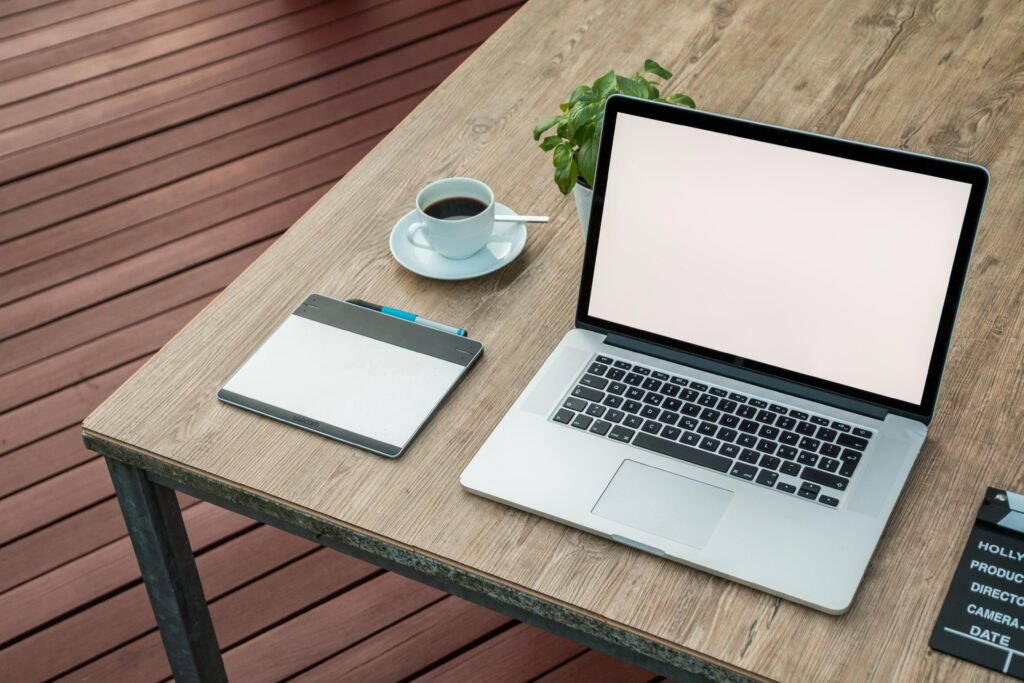
In today’s data age, having a thorough grasp of data consistency will be your differentiator. This knowledge will not only help you ace interviews but also help you in your career advancement.
Conclusion: Your Next Steps
Data consistency is the key to success in data science. You’ve now learned the key concepts, from what is data consistency to the differences between data consistency and integrity. Your journey to mastering these concepts has just started, and this knowledge will be your guide in your career. Now is the time to fearlessly tackle any data consistency questions that come your way!
This blog post has been designed as your guide to data consistency. We have covered everything ranging from basic definitions to practical usage and best practices. We hope this total understanding of data consistency has proven useful for career development!
And now for the secret message: As a show of gratitude for your efforts to read this complete guide to data consistency, you’re invited to join our premium Telegram channel! To get an invite, kindly comment below with your Telegram channel username, and we will send you an invite link. Our premium channel is a vibrant community of data professionals eager to guide and mentor each other. We also exchange insights, jobs, and more. You will indeed realize the value of your effort by joining us!
We welcome you to join our huge network of Telegram channels offering a treasure of useful resources and opportunities in AI, ML, Data Science, and Cybersecurity. We also have focused job notification groups, where we post the most recent jobs instantaneously to aid you in career growth in the fast-paced tech sector. Our groups are an invaluable resource to remain ahead of the curve and obtain opportunities speedily.
Lastly, we’d like to highlight the importance of these focused data science internships. Our focused Telegram channels and job notification groups frequently upload internships so that you may gain hands-on experience and skill development. This hands-on experience is invaluable to land a dream job.
2 thoughts on “What is Data Consistency vs Integrity | Here is the best guide”