Introduction
Hey all, I am a data scientist and AI enthusiast in India. Do you have an interest in furthering your career in data analytics? We offer lucidation on the limitations of linear regression, which includes how it can be misinterpreted to achieve success. We will explore the reasons behind the failure of linear regression in regression analysis and provide advice on how to tackle interview questions confidently and with confidence… With a cup of tea and chai, you can get ready for your interview and career transition. (Why Linear Regression Fails)
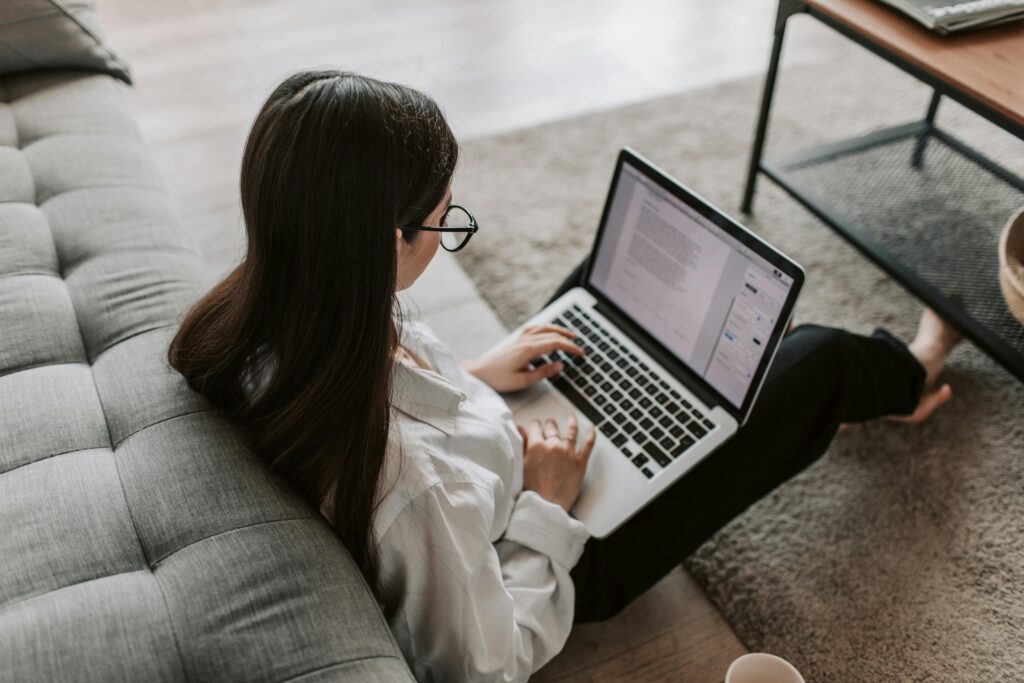
We will examine the potential dangers of Linear Regression in Python, R, and other programming languages with real-life scenarios. Acquiring these flaws is crucial for any data professional. These tips are highly recommended. Learn more about them here.
This final edition focuses on the most common reasons for the failure of linear regression in real-world applications, especially using Python and R. It also provides an introduction to the theory behind it, along with examples from India and abroad that are easy to understand by Indian students and working professionals. We will also cover some important details in Excel and other tools so that you can avoid costly mistakes in your regression analysis as well. With this book, you’ll learn why Why Linear Regression Fails and the fundamentals you need to know in order for data science.
Although linear regression can be useful in data analysis, it is not without its drawbacks. To gain insight into these issues, we will examine “Why linear reconstruction fails in R“, “why linear model regression fails vs Excel?”, and finally “What is the reason for linear residual error in Python“ to provide guidance on how to tackle them effectively. You’ll benefit from this thorough examination, enabling you to analyze complex data sets with confidence and construct more accurate models. We will provide a detailed analysis of “Why Linear Regression Fails.”
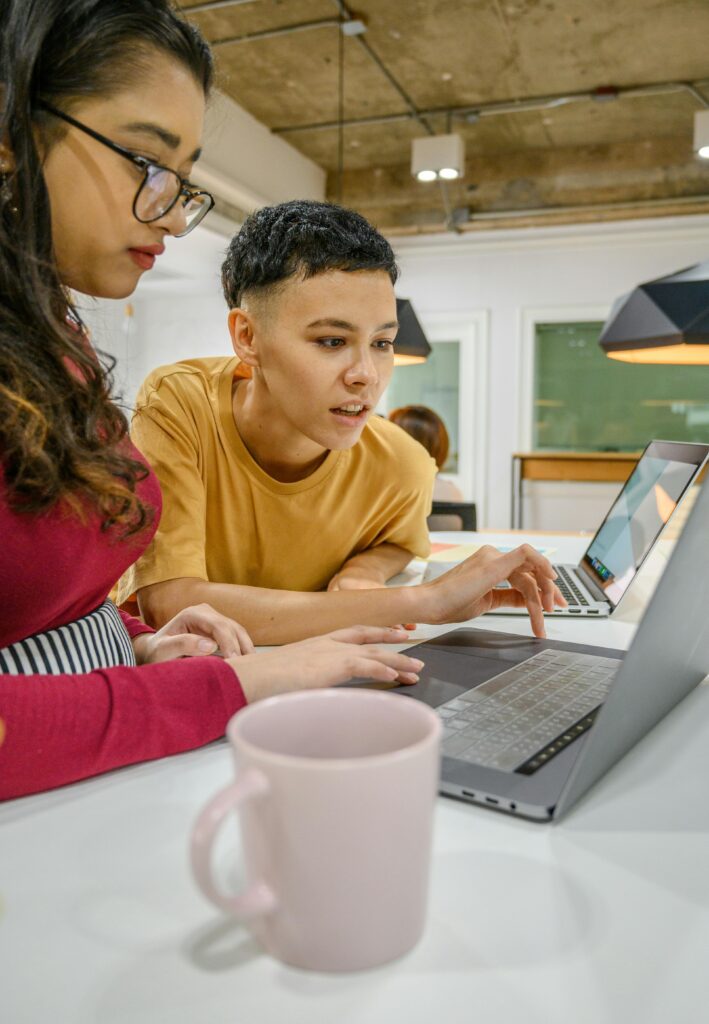
Understanding the Limits of Linear Regression
The fundamental concept of linear regression in statistical analysis is the assumption that variables are linearly related, but this assumption is not always accurate. In certain situations, the method may not be effective. We’ll examine these issues and provide guidance on how to identify them. What are the potential complications?
Non-linear Relationships
Non-linear relationships between the dependent and independent variables are often the reason why linear regression is not effective.
When this occurs, the data’s trend will not be a straight line. The complexity of the situation can be captured better through alternative modeling methods.
Outliers
Values that significantly differ from the rest of the data are known as outliers. They can severely distort the regression line, resulting in a flawed model. Additionally, there may be outliers in implementations of “Why Linear Regression Fails in Excel.” One of the critical skills to acquire is the ability to recognize and handle outliers.
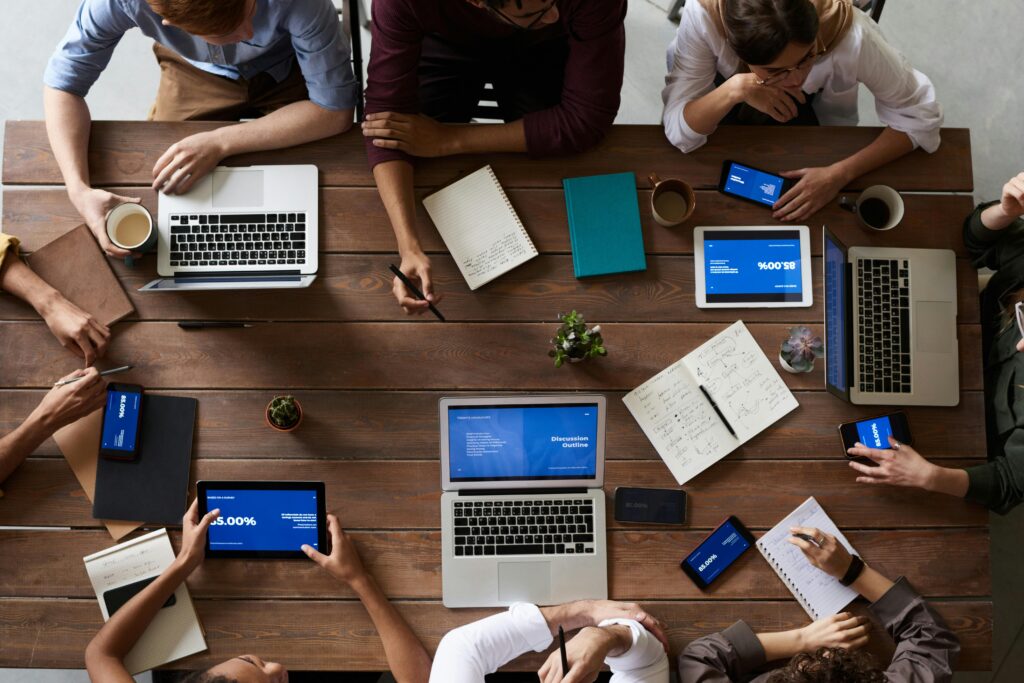
Multicollinearity
Independent variables are strongly related to each other. The dependence of dependent variables on each variable can be misleading and difficult to interpret, as seen in “Why Linear Regression Fails in R.”
Heteroscedasticity
The occurrence of heteroscedasticity is due to the fact that the variance of error terms differs between different values of independent variables.
This assumption is necessary for reliable confidence intervals and accurate standard errors; ignoring heteroscedasticity can result in incorrect inferences in regression models, as demonstrated in “Why Linear Regression Fails in Python.”
Missing Values
Incomplete information may affect the accuracy of regression analysis. Missed data can occur in regression analyses using Excel and Python. Proper handling of missing values is also important. Why?
Influential Points
Excessive values that have a disproportionate effect on the regression line are known as influential points, similar to outliers. The model’s fit and interpretation in regression analysis and its outcomes can be significantly impacted by them.
Small Sample Size
A small sample size can make it difficult to generalize the model to a larger population.
This is particularly relevant when working with large datasets and implementing regression models in Python.
Taking Stock of the Losses and Progressing Forward
By recognizing these limitations, you will be capable of identifying and managing potential failures. This knowledge is crucial for the creation of strong and reliable models.
Let us provide you with some advice on how to solve these problems. We’ll explore how these issues are addressed in various data science domains, including Python programming languages like R and Excel. With this comprehensive guide, you can tackle practical data challenges and confidently navigate interviews.
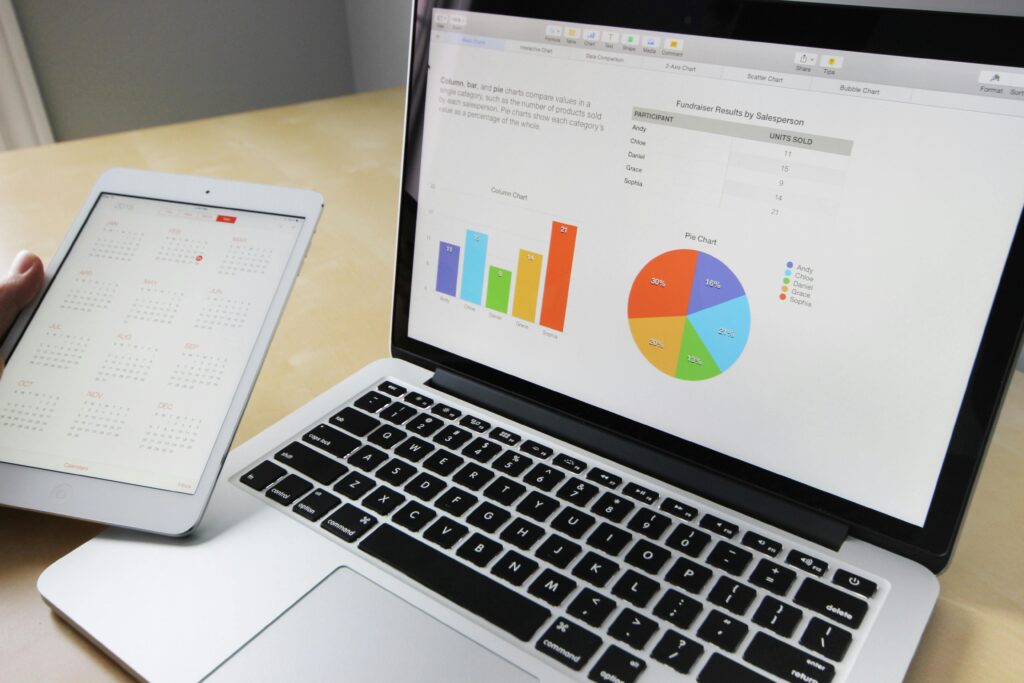
Conclusion
By utilizing “Why Linear Regression Fails,” you have learned the fundamental principles of data science modeling. You are now equipped to recognize and tackle the drawbacks of linear regression regardless of the software used. This book provides insight into why linear calculus is flawed. Send us a private message to gain access to our premium Telegram group by entering your channel name and receiving an invitation. It’s a fantastic group of data enthusiasts, offering free access to internship data science job alerts and related information.
This blog post aimed to address the common reasons behind linear regression’s failure and the practical implications of these issues, offering solutions and insights into how to handle these scenarios. By understanding the factors that can lead to inaccurate or unreliable results in linear regression, you can significantly improve your data analysis capabilities. This blog post has provided a detailed and insightful approach to “Why Linear Regression Fails,” offering actionable strategies and practical techniques for enhancing your modeling skills.
In closing, this deep dive into “Why Linear Regression Fails” should equip you with the knowledge to identify and address these potential pitfalls, leading to the development of more robust models in regression analysis. We’ve worked hard to provide you with a complete and thorough understanding, and we encourage you to leverage this valuable knowledge as you continue on your data science journey.
Join our Telegram Channels for more!
Our Telegram community offers a wealth of resources and support for aspiring data scientists. We regularly post job notifications and valuable data science tips, alongside internship opportunities in the field. It’s your one-stop hub for boosting your data science career.
Join our dedicated job notification groups – filled with internship-related data science opportunities, interviews, and more! And don’t forget, comment below with your Telegram channel name for an invite to our exclusive premium Telegram channel, where you can connect with a supportive community of like-minded individuals. Let’s continue this data journey together!
3 thoughts on “Why Linear Regression Fails | Best Guide to for Linear Regression”